If your business is caught up in endless—and sometimes confusing—discussions around why, how, where, and when to adopt Generative AI, take heart. You are having the right discussions. Sooner rather than later, your organization will have taken major leaps with Generative AI. Gartner says that over 80 percent of enterprises will have used Generative AI APIs, models, and applications in production environments by 2026. A Gartner study also found that enterprises with pilots in Generative AI had risen from 15 percent in March-April 2023 to 45 percent by October 2023. Another 10 percent were already in production mode. The race to get ahead by infusing Generative AI into business and IT functions is well and truly on. Is there a way to make the race go well? There is: If the Generative AI pilots and in-production initiatives are aimed at automation, analytics, and applications, the investment will likely deliver visibly fast ROI. The three domains—automation, analytics, and applications—form the 3A Axis around which most enterprises should place their initial bets.
Where and How to Apply Generative AI: The 3A Axis
Automation: The first mile in automation has been run by most enterprises. Simple, everyday tasks have been automated, eliminating manual labor and improving workforce productivity. These are tasks like employee onboarding, clearance, settlements, placing orders, generating reports, transferring data, using computer vision to scan products for quality assessment, etc. Generative AI is now being used to extend the automation footprint to knowledge automation. Some examples of knowledge automation include using large language models (LLMs) to contextualize and interpret enterprise knowledge, such as summarizing legal proceedings, an insurance claim, or customer feedback. It could even help create new knowledge or new content. For example, it could be used to provide new incident mitigation processes or turn customer reviews of a product into a story. The story could then be given a creative visual interpretation by Generative AI tools, in effect creating content that did not exist.
Analytics: A new paradigm in the consumption of analytics is arriving in the wake of Generative AI. Until now, traditional data warehouses have been trawled, and various tools—regression analysis, Monte Carlo simulations, etc.—have been applied to the data. The output is tables, graphs, charts, diagrams, histograms, maps, box plots, etc. Now imagine a marketing manager asking a simple question, in plain English, using Generative AI, “What is the sales trend in EMEA?” or a product manager asking, “Why do customers hate my product?” and getting an answer in plain English. It changes how analytics is consumed and, more importantly, changes who can consume it by removing the technical literacy required to consume analytics. In truth, the powerful capabilities of analytics are unlocked by an ability to ask the right questions. In a Harvard Business Review article, researchers Hal Gregersen, Senior Lecturer in Leadership and Innovation at the MIT Sloan School of Management, and Nicola Morini Bianzino, Global Chief Technology Officer of EY, say their studies showed that 94 percent of the time, AI-led respondents had questions that differed from non-AI-led respondents. This means Generative AI can help non-technical users in asking more abstract questions, shifting the focus of analysis from identification to ideation.
Applications: Over the last decade, AI has been steadily used to improve enterprise applications, ensuring a more consumer-style experience with intuitive and accelerated workflows. Generative AI has set this trend into turbo mode. SAP has Joule, a natural language processing (NLP) Generative AI copilot, embedded in its HR, finance, and supply chain applications. Joule lets users chat with the enterprise data and ask questions in plain English, such as, “How many sales orders were canceled last year?” Salesforce has Einstein, a Generative AI assistant, for its CRM application. ServiceNow has Now Assist, which leverages Generative AI to speed up app delivery. The user describes the process flow and Now Assist authors the code.
The changes triggered by Generative AI in automation and analytics can be best harnessed through a CoE. Today, the basic foundation blocks of Generative AI, like data, analytics, knowledge models, LLMs, NLP, and neural networks, are housed with the data science team. Interestingly, we are seeing an integration of data, analytics, and automation CoEs.
Most large organizations have an enterprise-level data and analytics CoE to further the cause of data democratization and unlock analytics' value. These organizations have also created CoEs for automation. However, the boundary of automation has moved to knowledge-based domains.
Today, knowledge-based tasks are getting automated using Generative AI. A simple example is a Generative AI-based research assistant that lets legal experts converse with massive libraries of digitized legal information. Marketing teams can create text, audio, images, and video using text prompts with ChatGPT, Audiogen, Stable Diffusion, and Synthesia.
Organizations need to extend their traditional data, analytics, and automation CoEs without setting up a new one to stay on top of these developments and leverage them. They can do this because Generative AI uses the same tools and skills. These tools can be merged to create a Generative AI CoE.
The Challenges of Applying Generative AI
The advances in Generative AI are not without hiccups---some of which revolve around the ethical use of data, loss of fidelity, and nonsensical/hallucinatory outputs, and can be inordinately frustrating and challenging. Additionally, there are caveats related to cost and practicality. Large Language Models can be expensive, difficult to maintain, and lead to ethical conflicts. Therefore, in several instances, organizations will do well to train their Generative AI models on data owned by the organization instead of relying on publicly available data. Aside from eliminating anxieties around public data sets, using internal data also produces early success with Generative AI. Over the last six months, our experience has shown that when internal data is used, the fidelity of the results is high.
Our experience also shows that marketing agencies use Generative AI for images more effectively than IT service providers. Image generation tools are maturing rapidly. Marketing agencies find integrating these tools into their existing workflow and traditional toolsets easier than a BPO. By contrast, audio and video tools are not as mature and have inherent problems like deep fakes and IP violations.
From Pilots to Production, Securely
We know that Generative AI pilots have taken off, and experimentation has begun. However, few organizations have built a Generative AI CoE that can scale up their initiatives and ensure consistency of results in production. But organizations know they must put in place a strategy for Generative AI adoption so they can move from pilots to production and quickly scale their initiatives for broader adoption across the organization. The strategy must include two vital components: data privacy so the organization can enjoy public trust, and compliance requirements that governments are quickly implementing.
The reality is simple—but daunting. The technology is evolving rapidly; the jump from, say, a ChatGPT 3.5 to ChatGPT 4.0 will not be incremental—it will be steep, sharp, and demanding. The tools will be expensive. Identifying the right use cases to deliver ROI will be difficult. And the ethical challenges will be a headache. At this point, organizations need frameworks and assessments to navigate the technology and help make the right decisions. And it is the decisions that are taken wisely, with the insights and expertise of technology partners, that will help create the lightning rods for Generative AI within the organization.
In the article, the authors narrate how Colgate-Palmolive differentiated their products with charcoal using AI to generate less apparent questions when querying data from a 20-year history of charcoal use in South Korea, putting the company ahead of the product curve by a decade.
These CoEs moved from RPA to intelligent process automation and then to cognitive tasks such as OCR, IDP, and conversational agents—stringing together an intelligent automation toolkit
About the author
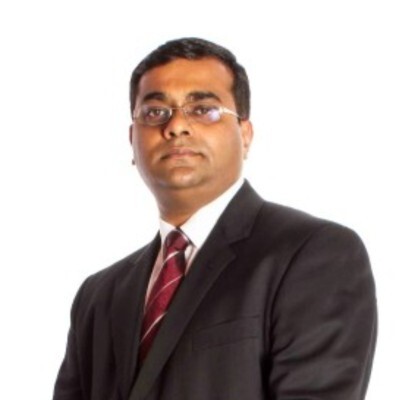
SVP and Head, Business Consulting at ITC Infotech
Sandeep brings over 28 years of leadership and consulting experience in management consulting and manufacturing operations. He has effectively led multiple service lines, managed P&L, and made significant cross-industry impacts in areas like manufacturing, consumer goods, retail, and hospitality. His extensive global experience covers the Americas, Europe, and Asia-Pacific. Sandeep's skills in business process re-engineering, business analytics, and program management have been instrumental in driving technology-led business transformation journeys for clients across various global markets.