As technology progresses, astronomical data creation is inevitable. According to a report by Statista Research Department, global data creation is projected to grow to more than 180 zettabytes by 2025.
With so much data being generated, it is challenging for businesses to organize and derive insights from the sea of unorganized data. It is where cluster analysis comes to the rescue. Cluster analysis can help us sort objects into different categories by identifying similarities and differences between different objects.
Cluster analysis is often used as a preprocessing step to identify patterns in data relevant for further analysis and interpretation. In other words, it aims at exploring and analyzing patterns from data samples and dividing them into broad groups. You can use cluster analysis for various purposes, such as reducing datasets’ dimensionality (number of attributes) by grouping similar items. It helps simplify the analysis and makes it more efficient.
As identifying patterns in data using AI solutions can lead to new opportunities or previously hidden trends, businesses nowadays consider cluster analysis a powerful tool to aid their business decisions. In this guide, we will first understand what cluster analysis is and then cover various types of clustering, their requirements, limitations, and applications for your business.
What is Cluster Analysis Technique?
Cluster analysis is a data analysis technique for exploratory studies in which you can assign different types of entities to groups whose members share similar characteristics. Simply put, cluster analysis is discovering hidden relationships within massive amounts of data without detailing these relationships.
Cluster analysis enables you to sort the given entities into natural groups. The degree by which these entities are associated is maximum if they belong to the same group and minimum if they do not. You can then visualize the data structure as a multidimensional map in which groups of entities form clusters of a different kind.
Cluster algorithms in data mining are often shown as a heatmap, where items close together have similar values, and those far apart have very different values. It makes it easy to identify elements that stand out as outliers from the rest of the dataset.
9 Most Common Types of Clustering
Cluster analysis is subjective, and there are various ways to work with it. As more than 100 clustering algorithms are available, each method has its own rules for defining the similarities between the objects. Let us explore the most common ones in detail below:
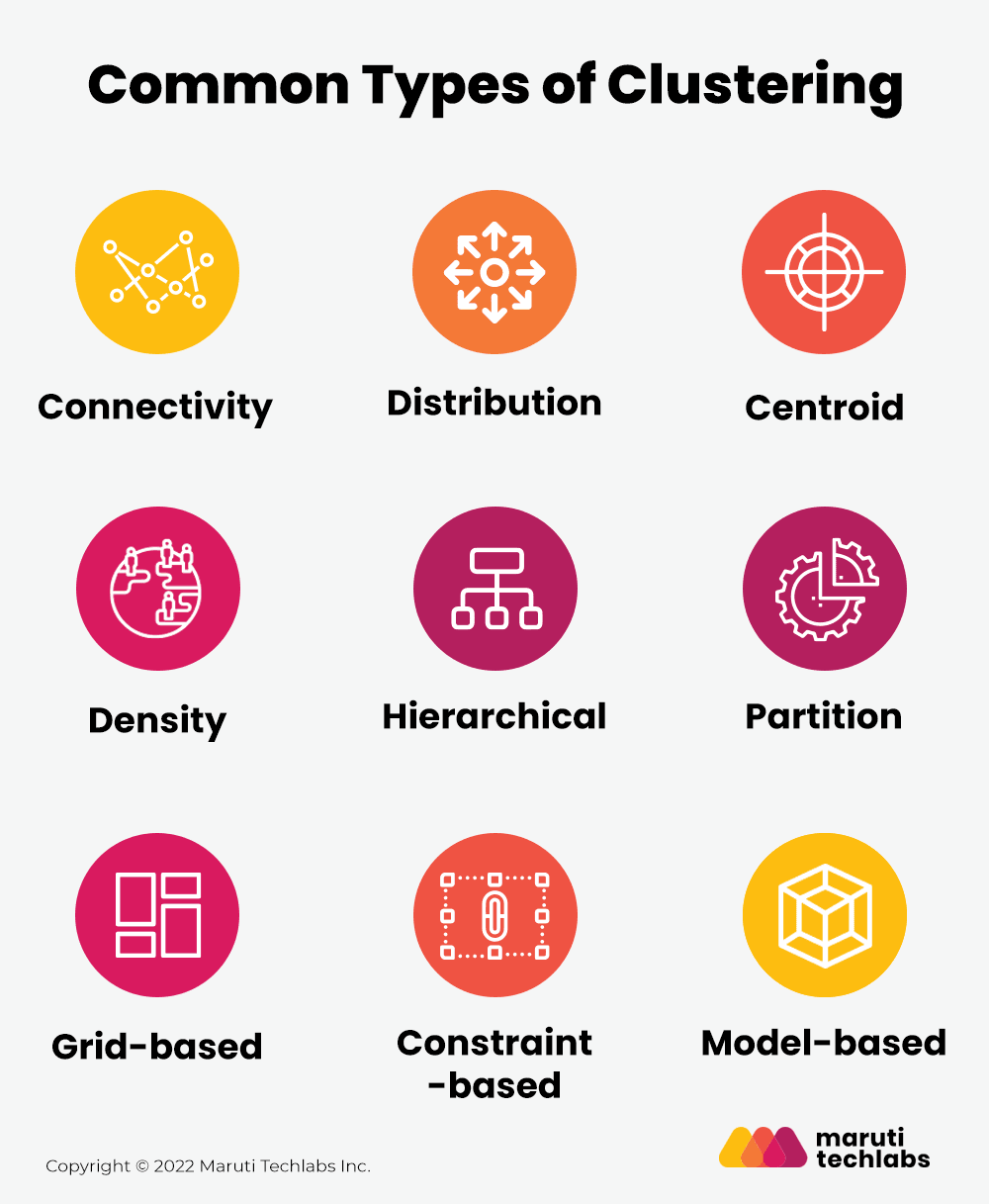
1. Connectivity Clustering
Connectivity models are where the data points closer in data space are more similar than data points farther away. You can further divide the connectivity model into partition-based and proximity-based models.
Proximity-based models use different functions for defining distance, though this is subjective. Partition-based models follow two approaches: the first approach involves classifying all data points into clusters and aggregating them as distance decreases. The second consists of all data points identified as a single cluster and partitioned as the distance increases. Proximity-based models are easier to interpret yet have a limited ability to scale for large datasets.
2. Distribution Clustering
In this type of cluster analysis, clusters are separated by the areas of density higher than the rest of the data set. Hence, the cluster is usually divided by the objects in sparse regions. Typically, the items in these light regions are noise and border points in the graph.
3. Centroid Clustering
It is an interactive clustering algorithm where the similarity is considered the proximity of the data point to the cluster’s centroid. K-Means cluster analysis is an example of a centroid clustering model, where k represents the cluster centers and elements are assigned to the nearest cluster centers.
When it comes to centroid models, the number of clusters necessary after the centroid model must be established, making previous knowledge of the dataset essential.
4. Density Clustering
This cluster analysis model is based on the density of the element. For instance, there is a lot of density when there are multiple elements adjacent to each other. Hence, those elements are considered to belong to a particular cluster.
Here, you can use a formula to determine the density of acceptable elements for a particular collection of information. If the computed density is less than the threshold, the collection in question has too few relevant elements to form a cluster.
5. Hierarchical Clustering
Hierarchical cluster analysis is a model that creates the hierarchy of clusters. Beginning with all the data points allocated to their respective cluster, the method combines the two closest clusters into the common one. At last, the algorithm will only stop when only one cluster is left.
Hierarchical clustering is further divided into two sections:
- Agglomerative Approach: Bottom-up approach combines the small clusters until all the groups merge into one cluster.
- Divisive Approach: Top-down approach where a cluster splits into smaller clusters in continuous iterations.
6. Partition Clustering
Assume you are given a database of “n” objects and the partitioning method constructs “k” partitions of data. Note that the partitioning approach may construct one or more partitions, with the number of partitions being fewer than or equal to the total number of objects in the dataset.
The following conditions must be satisfied by each data group:
- Each must contain at least one object.
- Each object must belong to just one group.
7. Grid-based Clustering
All the objects are combined in this cluster analysis to form a grid-like structure. The object space is then quantized into a finite number of cells to produce a grid structure. The most significant benefit of this clustering is the short processing time, as the cell density in each dimension of the quantized space does not affect this operation.
8. Model-based Clustering
This technique postulates a model for each cluster to discover the best data fit for that particular model. This approach locates the clusters and reflects the data points’ geographical dispersion by grouping the density function.
Model-based cluster analysis is one of the reliable clustering approaches. It allows you to automatically identify the number of clusters depending on the conventional statistics and accounting for outliers or noise.
9. Constraint-based Clustering
This cluster analysis technique executes the algorithm based on user or application-oriented constraints. A constraint is the user expectation or the attributes of the expected clustering results. Note that the user or the system must specify the constraints here.
Requirements for Cluster Analysis in Data Mining
Below are some of the criteria that clustering should fulfill in the data mining process–
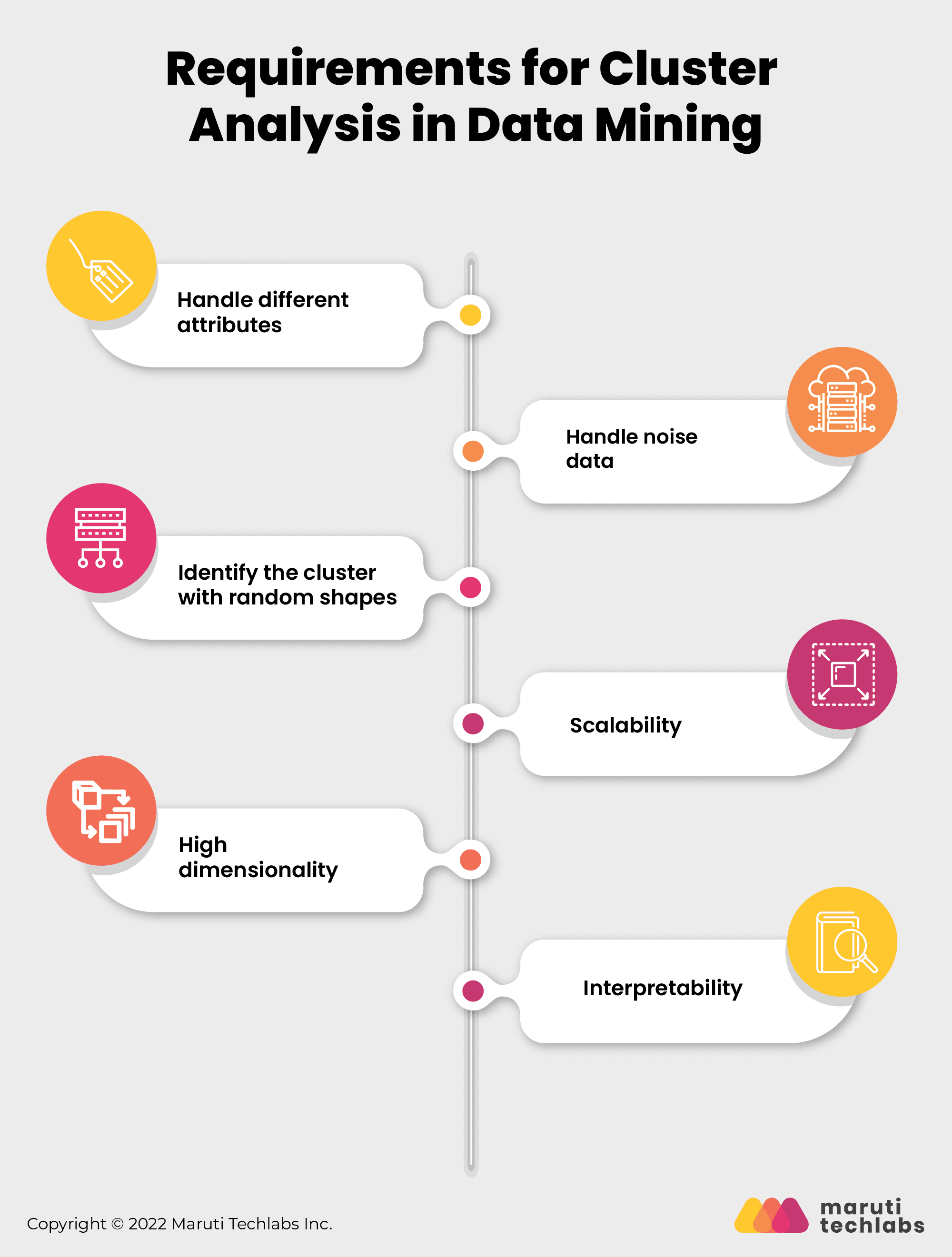
1. Handle different attributes
As a single cluster analysis algorithm may be used against multiple data sets with various characteristics, it is advisable to have a flexible clustering algorithm that can deal with multiple attributes like binary data, numerical and categorical data, etc.
2. Handle noise data
Datasets sometimes may contain irrelevant, missing, or noisy data. Several algorithms are sensitive to such data and may produce low-quality results.
3. Identify the cluster with random shapes
Clusters of any form should be detectable by the cluster analysis technique. They should not be restricted to distance measurements that locate spherical clusters of tiny sizes.
4. Scalability
When dealing with large datasets, it is necessary to have a highly scalable cluster analysis algorithm.
5. High dimensionality
Some datasets are low dimensional, and some are high dimensional. The cluster analysis algorithm must be able to handle both kinds of dimensionalities.
6. Interpretability
The result of the clustering algorithm must be easy to interpret and understand. Also, it is not possible to have new clustering algorithms for every data analysis. Hence, it helps to have an algorithm that is reusable to a certain extent.
Business Applications of Cluster Analysis
Every industry deals with tons of data. And where there is data, there is categorization. Clustering helps in the broad classification of data and hence has several business applications in today’s age. Let’s discuss some of them below:
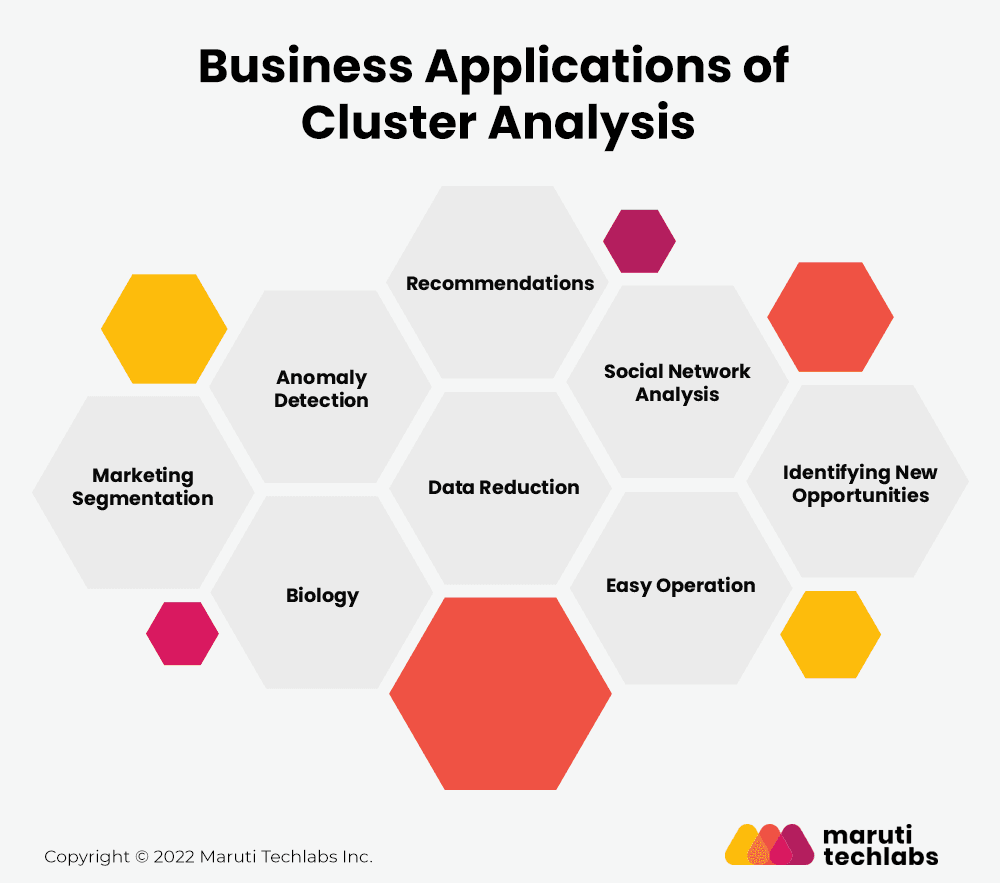
1. Marketing Segmentation
Cluster analysis techniques help marketers and companies divide their target audience into distinct segments with similar interests and features rather than having homogenous groups of consumers. Doing this helps businesses strategically target their products and services to those looking for the same.
2. Anomaly Detection
Cluster analysis in data mining is the best way to analyze anomalous activities, for instance, identifying fraud transactions. Cluster detection methods first examine the sample of good transactions and identify patterns, sizes, and shapes of regular activities. In case of a fraud transaction, the cluster will differentiate its pattern from a standard action and flag the activity.
3. Biology
You can use cluster analysis algorithms to develop plant and animal taxonomies, classify the genes with comparable functionality and obtain insight into population structures.
4. Identifying New Opportunities
Using cluster analysis for brands and products helps identify similar competitive markets with the same services or products. Further, it also aids in market research, pattern recognition, data analysis, and image processing functionality which can help improve business decisions. With these results, organizations can analyze their current growth relative to their competitors to identify the potential of new products.
5. Data Reduction
Data reduction is an undirected cluster analysis technique used to identify the hidden patterns within the vast data without formulating a specific hypothesis. To do the same, you may consider many clustering methods and choose the one which best suits your business requirements.
6. Recommendations
You may have got must-watch notifications from Netflix. Ever wondered how they know your taste in movies? The answer is cluster analysis. Cluster analysis enables recommendation engines to understand your preferences and provide you with something of your choice from the clusters of different genres.
7. Social Network Analysis
Social sites like Facebook and Instagram use clustering techniques to group people with similar interests and backgrounds. Doing this helps them show similar feeds to those of the same interest.
8. Easy Operation
Cluster analysis helps divide the extensive complex dataset into smaller parts and perform efficient operations. For example, you can improve the results for logistic regression by performing operations on smaller clusters that behave differently and follow different distributions.
Validation of Cluster Analysis
Once you are provided with the results for your cluster analysis, it is time to validate your results. But the question is, how? Let’s try to find the answer using two ways of validation of cluster analysis:
1. Internal Validation
Use alternative predictive analytics methods to compare the outcome of cluster analysis quantitatively. Note that this does not guarantee that one or other method is the right one; it merely illustrates some potential options! This way, we can know which approach yields the best results and which you might want to consider using to organize your data further for more convenient analysis.
Internal validation further includes three measures of validation discussed below:
- Compactness: This measurement helps identify how close the elements are within the cluster. Note that the different indices for evaluating the cluster’s compactness depend on the average distance between the observations. As a result, the lower within-cluster variation indicates good compactness and vice versa.
- Separation: This parameter helps indicate how well the cluster is separated from other clusters. The indices used for separation measures include the distance between the cluster center and the pairwise distance between different elements inside the cluster.
- Connectivity: This parameter specifies how closely objects in the data space are clustered with their nearest neighbors. The connectivity ranges between 0 and infinity and should be kept as low as possible.
2. External Validation
To test the validation of your cluster analysis algorithm, you can apply it to another dataset whose outcome has been already determined. This approach can have disadvantages as well! The test set may have been put together in a way that suits one method better than the other.
Overall, it means that validation for clustering doesn’t show us exactly which methods are better or worse for specific data sets, but they still may be considered valuable. Absolute value cannot be attached to a validation method.
What are the Limitations of Cluster Analysis?
The biggest drawback of cluster analysis is that the term “clustering” is a broad-ranging term. It means that there are various methods to segregate data into groups. Consequently, different ways of clustering yield different results. This happens because different ways of grouping are based on different criteria.
Also, there are many cases where you are unaware of whether the chosen cluster analysis technique is relevant to the given problem or not. Therefore, another limitation of cluster analysis is that there are minimal ways by which you can validate the results you obtained.