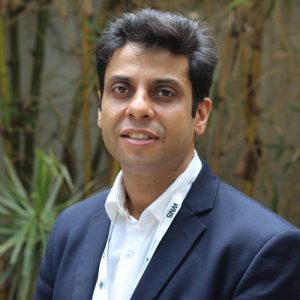
By Nitin Sareen, SVP-Group Data & Analytics, Aditya Birla Group
A recent article (https://www.theregister.co.uk/2019/03/05/eu_startups_no_ai/) talks of a mega survey of ~3000 startups in EU that revealed that two in five ‘AI startups’ essentially have no AI.
Surprising? May be not. As the article points out, “Fad-obsessed VCs are pouring money into AI and blockchain, so startups tune their pitches accordingly.” While it is easy to stick the “AI Inside” label and attract funding, it is not as easy to truly becoming an AI-driven company.
Having seen it at a few places and being in the midst of the journey at my current organization, here is my take on what it takes to becoming an AI driven (or data driven, I may say) organization:
1. Culture: Data Culture is Decision Culture
As philosophical or cliched it may sound, it is critical to have the culture of being led by data right from the top and instil the same deep down in the organization. Over time, astute leaders develop a sense of their business numbers and are comfortable taking decisions based on their gut feel. It works, but may not be scalable. Using data to guide the decisions is important to be practiced at the top.
At the same time, don’t approach AI as a cool “science experiment” or an exercise in amassing data for data’s sake. The fundamental objective is collecting, analyzing, and deploying data to make better decisions.
While doing that, it is very important for leaders to stay true to the business problem: What is it and how can you solve it? Simply relying on having huge quantities of data in a data lake is not enough. For many leaders the inclination is to say, “OK, I have lots of data. What insights can I get?” There is no shortage of data, and there is even more data coming in. This approach won’t take us too far. Starting from the business outcome and saying “OK, for this outcome, let’s look at the landscape of data. What kind of insights do I need and how can AI help?” is a better approach.
Commitment from the CEO and the board and having AI initiatives driven from the top of the house is essential. It must be the CEO, or her direct report, who understands what AI is and what it can do, who should take accountability of driving AI in the company. Anywhere below this, it either gets kicked around or gets too focused on the function(s) it belongs to and finds lesser acceptance at the enterprise level.
2. Capture, process and democratize data
In Harvard Business Review’s words – if your company isn’t good at data, then it’s not ready for AI.
A key driver to enable the culture is to make it easy for all the teams to capture and consume all of the data in the organization. An enterprise has a plethora of data sources, both internal and external. These can range from different business applications, product applications, public and private customer interaction points, monitoring systems, third-party data providers, and many others. These systems are generally set up for operational reasons, with collecting data for analytics being an afterthought. As a result, the valuable data from all of these sources continues to remain in its silo. This leads to many lost opportunities of deriving insights or optimizations by putting data from different sources together.
Few steps necessary to enable this are:
- Take an inventory of all the data sources and create a common data-capture infrastructure that is standard across the company and that lays out the correct way to capture and log the data.
- Consolidate and process all of the data so that all consumers of data in the organization know where to go to find it. Some call it the Data Fabric. With standardization of capture and consolidation of data, it becomes very easy for the AI team to access the data and accelerate the pace of generating better insights for strategic decisions.
- While doing this, good data governance and privacy are paramount to ensure the quality of data is maintained.
- Democratization of data with regulated access helps people to believe in it and to deliver solutions. It also creates a great deal of transparency. When everyone sees and starts believing in the data, it’s a game changer: they begin to change their behaviours & business processes. It acts like oxygen!
- Cloud based platform that provides elastic capacity for storage and processing is a must for a large enterprise. This allows one to beat the “too much or too less” paradox of infrastructure investment upfront. Along with the platform, the teams need to have access to best in class tools for processing, analysing, visualizing data.
3.Software and tech ? AI
Yes, I want to make it very clear. Just building a tech layer with data storage and building a software solution on top of it is NOT the same as building AI. The models that power the intelligence is not trivial and do not treat them so. I have come across many companies where the focus is mainly on building a fancy and shiny technology wrapper that looks good and there is no real AI in the solution.
True story: a friend of mine told me that their company launched a customer facing chatbot, but it did not have the right AI powering it. They then needed an army of people typing away the responses! That is why the result of the survey is not surprising.
Software is important and equally important are the Models. There are few individuals who are equally conversant with both. Unless there is a team that is full of such unicorns, getting the right talent mix is paramount. AI is a team sport and it is important to have the right team to play the game. Some distinct skills needed are:
Data engineers
Responsible for getting data into the platform so that the data scientists and analysts can get to it. They are responsible for providing mechanisms to capture data from different sources in the organization including end products. They make sure that the data is available, curated, and cleansed. They’re generally a part of IT, reporting to the CIO.
Data scientists
Involved in statistics, machine learning, and deep learning. They rely on advanced data mining tools for putting together predictive and prescriptive analytics. Conversant with R, Python, and Scala they possess deep understanding of machine learning toolkits. They tend to have a strong mathematics background and report to the Chief Analytics Officer.
Data analysts
Typically embedded in line-of-business or functional groups. Their job is to transform business questions into queries on the available data sets.
They might not fully understand the inner workings of AI algorithms, but they can apply some of them to the questions they are trying to answer.
Line-of-business users
The ultimate users of the data to make decisions. They are provided with insights, ad hoc analysis tools, etc. These are the people that take analysis and act on it; for example, in the case of marketing, campaign managers might look at the ROIs of certain campaigns and decide to move their budgets accordingly.
4.Experimentation – prototyping & working with start ups
For accelerating the path of adoption for AI driven insights, an experimentation mindset helps. Recommendations form hypothesis which need to be tested and validated. The process to follow is:
Build a clear hypothesis: What’s the specific theory or idea being tested? What would you consider to be a successful outcome? As you’re building a hypothesis, make certain you’re being rigorous in keeping it user-focused.
Define the success metrics: What are the very specific, most relevant metrics to use, and what outcomes would prove its feasibility or value?
Test the hypothesis: build a working implementation to demo and the test framework for measuring results.
Learn and iterate: Measure the results & brace yourself for the very likely chance your experiment results in no meaningful change in metrics. That’s a key learning in itself.
Another way to accelerate the journey is to experiment working with start-ups that have created sharp solutions. Understand that they would not be ready for completely scaling up and may also not solve for the entire puzzle. Most often they provide a solution for a small piece of the puzzle. It can be a good starting point that can give a head-start and build the rest of the pieces along with it.
5.Value
Predefining a value discovery framework to identify and benchmark the levers that would be affected by AI helps in focusing on the right KPIs. It also helps aligning the efforts to the important priorities of the business, namely revenue growth, cost reduction and/ or enhanced customer satisfaction.
Measuring the success metric after the recommendations have been incorporated in the business process and comparing it with the benchmark gives the incremental return from the analysis.
While this may help determine the direct impact, there are multiple ancillary benefits that accrue to the business by enhanced processes & governance. The intangible value of understanding the emerging trends that are apparent from the data and changing business strategy and decisions make the value of AI even larger.
Last words: AI is not a magic wand and will not solve the business problems overnight. It takes time for the entire strategy to come to life, the data to become a strategic asset, for the AI teams to create impact and for the business to realize the value. It is a journey that takes time and for the orchestra to produce a symphony!