In the rapidly evolving landscape of technology, artificial intelligence (AI) has emerged as a transformative force, reshaping industries and redefining the boundaries of innovation. As organizations increasingly embrace AI to drive growth and efficiency, the demand for skilled AI project managers has surged. However, managing AI projects presents unique challenges that set it apart from traditional software development. This guide aims to illuminate the path for project managers venturing into the AI domain, offering insights into the distinctive aspects of AI project management and strategies for success.
AI Project Canvas
The adoption of AI across industries has been nothing short of revolutionary. According to McKinsey & Company's 2022 Global Survey on AI, the implementation of AI products and services more than doubled from 2017 to 2022. This trend shows no signs of slowing, with 63% of survey respondents anticipating increased investment in AI over the next three years.
However, the journey from concept to production in AI projects is fraught with challenges. A 2022 Gartner survey revealed that only 54% of AI projects successfully transition from pilot to production. This statistic underscores the need for specialized project management approaches tailored to the unique demands of AI development.
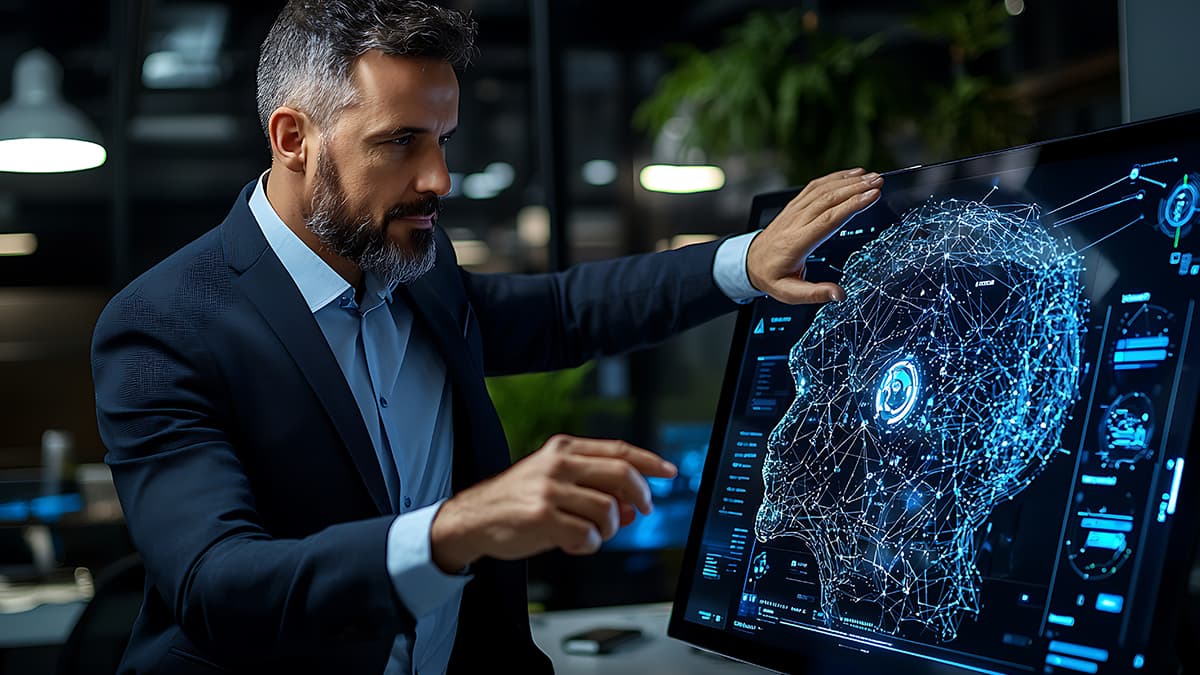
Key Differences in AI Project Management
AI project management diverges from traditional software development in several crucial ways:
• Data-Centric Approach: While traditional software projects focus primarily on code, AI projects revolve around data. The quality, quantity, and relevance of data often determine the success of an AI project.
• Iterative Model Training: AI models require repeated training and refinement. This iterative process means that progress is often non-linear and difficult to predict.
• Ethical Considerations: AI projects must navigate complex ethical terrain, addressing issues such as bias, fairness, and transparency.
• Interdisciplinary Collaboration: AI projects typically require collaboration between data scientists, domain experts, software engineers, and ethicists, necessitating strong communication and coordination skills.
• Uncertainty and Experimentation: The experimental nature of AI development means that outcomes are often uncertain, requiring a higher tolerance for ambiguity and failure.
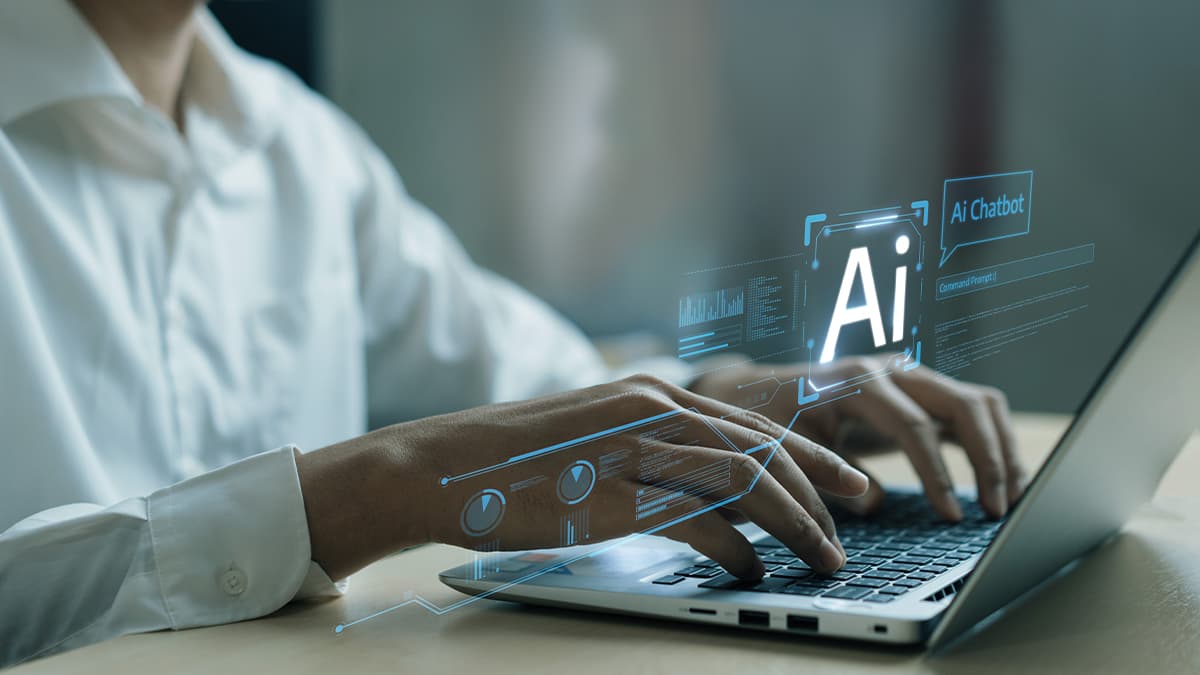
AI Project Life Cycle
Understanding the unique life cycle of AI projects is crucial for effective management. Let's explore the key stages:
1. Business Understanding and Problem Definition
The foundation of any successful AI project is a clear understanding of the business problem it aims to solve. This crucial stage involves identifying specific business needs, defining measurable objectives, assessing the AI solution's feasibility, and aligning stakeholders on project goals and expectations.
Key questions must be addressed to validate the project's direction. Is AI the most appropriate solution for this problem? What specific value will AI bring to the business? Are the necessary resources and data available?
By thoroughly examining these aspects, organizations can ensure that their AI projects are built on a solid foundation, increasing the likelihood of success. This initial stage sets the tone for the entire project, guiding decision-making and resource allocation throughout the AI development process.
2. Data Discovery and Preparation
Data is the lifeblood of AI projects. The data discovery and preparation phase is often the most time-consuming but crucial part of the project. It involves:
• Identifying and accessing relevant data sources
• Assessing data quality and quantity
• Cleaning and preprocessing data
• Addressing data privacy and security concerns
According to Appen's 2022 State of AI and Machine Learning Report, 41% of respondents cited data management as the biggest bottleneck in AI initiatives. Implementing a robust data management strategy is therefore critical.
3. Model Development and Training
This stage focuses on designing, developing, and training the AI model. Key activities include:
• Selecting appropriate algorithms and model architectures
• Splitting data into training, validation, and test sets
• Iterative model training and hyperparameter tuning
• Evaluating model performance against defined metrics
Project managers should work closely with data scientists to establish realistic timelines and milestones, recognizing that model development is often an exploratory process.
4. Model Evaluation and Refinement
Once a model is developed, it must be rigorously evaluated to ensure it meets the project's objectives. This stage involves:
• Testing the model on held-out data
• Analyzing model performance, including accuracy, precision, and recall
• Identifying and addressing biases or ethical concerns
• Iterative refinement based on evaluation results
It's crucial to involve stakeholders in this process to ensure the model meets business requirements and ethical standards.
5. Deployment and Integration
Deploying an AI model into production environment presents unique challenges. Key considerations include:
• Scaling the model to handle real-world data volumes
• Integrating the model with existing systems and workflows
• Implementing monitoring systems to track model performance
• Developing protocols for model updates and maintenance
A phased deployment approach, starting with a pilot and gradually scaling up, can help manage risks and ensure smooth integration.
6. Monitoring and Continuous Improvement
The work doesn't end with deployment. AI models require ongoing monitoring and improvement to maintain performance over time. This stage involves:
• Tracking model performance in real-world conditions
• Identifying and addressing concept drift or data drift
• Regularly retraining models with new data
• Continuously gathering user feedback and refining the model
Appen's report indicates that 91% of organizations update their machine learning models at least quarterly, highlighting the importance of this ongoing process.
Agile Adaptation for AI Projects
While Agile methodologies provide a solid foundation for AI project management, they often require adaptation to suit the unique nature of AI development:
1. Flexible Sprint Goals: Instead of delivering functional increments each sprint, goals might focus on data preparation, model improvements, or evaluation metrics.
2. Data Backlog: Alongside the traditional product backlog, maintain a data backlog to prioritize data acquisition and preprocessing tasks.
3. Extended Definition of Done: Expand the definition of done to include criteria such as model accuracy, bias checks, and ethical considerations.
4. Cross-Functional Teams: Ensure teams include a mix of data scientists, domain experts, and software engineers to foster collaboration and knowledge sharing.
5. Experimentation Sprints: Allocate specific sprints for pure research and experimentation to explore new approaches or technologies.
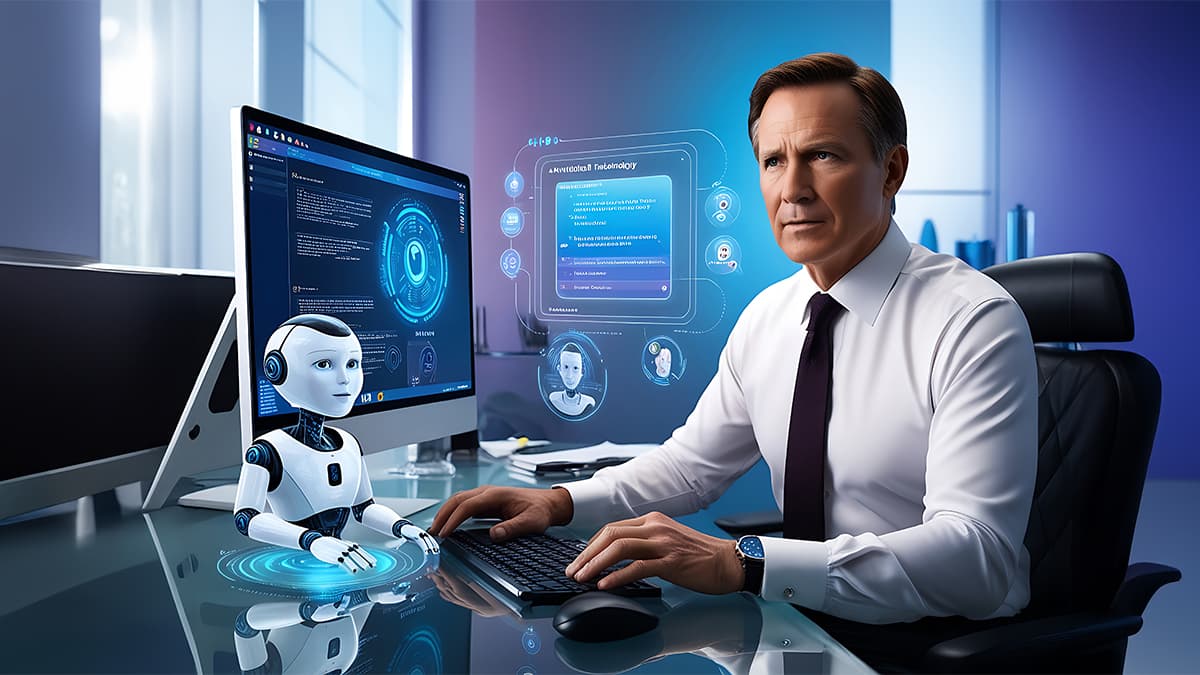
Ethical Considerations in AI Project Management
As AI systems increasingly impact people's lives, ethical considerations must be at the forefront of AI project management. Key areas to address include fairness and bias mitigation in AI models, as well as transparency and explainability of AI decisions.
Equally important are data privacy and security, along with accountability for AI outcomes. Project managers should integrate ethical reviews throughout the project lifecycle and consider forming an ethics advisory board for guidance on complex issues. By prioritizing these ethical aspects, AI projects can ensure responsible development and deployment of AI systems that respect human values and rights.
Project managers should integrate ethical reviews throughout the project lifecycle and consider forming an ethics advisory board for guidance on complex issues.
Managing AI projects requires a unique blend of technical understanding, business acumen, and ethical awareness. By recognizing the distinct characteristics of AI development and adapting project management approaches accordingly, organizations can navigate the challenges and harness the transformative potential of AI.
As the field continues to evolve, successful AI project managers will be those who remain adaptable, embrace continuous learning, and foster collaboration across disciplines. With the right approach, AI projects can move beyond the pilot stage to deliver real-world value and drive innovation across industries.
The article was first published on CSM Blog Named: Managing The AI Project Management Lifecycle