Everybody who nurses their inner geek would be familiar with JARVIS, Iron Man aka Tony Stark’s very own AI-enabled personal assistant. One of the most fascinating aspects of Jarvis was his ability to speak to Tony, understand his needs and provide fixes and solutions.
Modern life isn’t looking too different from the magic of the Marvel Cinematic Universe…at least from a technology perspective. Conversational AI is probably one of the biggest developments in the field of AI today, quickly transcending the boundaries of entertainment and leisure, and playing a critical role in shaping business insights, enabling governance and aiding the public with information.
One of the first companies in India to revolutionise the AI landscape is Haptik.ai, also among the world’s largest conversational AI companies having reached more than 100 million devices, processing more than 2 billion conversations. Haptik’s enterprise partners include Coca Cola, KFC, Future Group, Club Mahindra, OYO, Grofers, Viacom18, among others – these companies have converted several aspects of their service pipeline into a conversational model using the tools provided by Haptik.ai – namely an enterprise tool kit, a software development kit (SDK) and an ad-tech platform for engagement.
Recently, Haptik discovered that Mobile Consumer Engagement is a challenge faced by every business in the digital era. One of the main reasons for this is the oversaturation of the market. Given that the average smartphone user receives over 49.5 push notifications per day and more than 100 apps on their phone, with access to a catalog of 3M Android apps on Google Play, standing out among this multitude is a tall order.
While acquiring customers is fairly easier and cheaper, retention is a growing challenge for new apps in the market. Retention is a key metric, which determines an app’s success in the long run. Engagement determines how connected a user to the product or service. Therefore, good engagement rates justify a high loan to value ratio for a business as they promise to build on existing engagement rates and grow them.
Haptik’s clients wanted to boost engagement on their existing mobile platforms with an all-in-one service – which did not require major development efforts and without an exponential increase in their app’s memory footprint. They wished to leverage a medium that would increase their retention rates and provide great utility to their users. It was also necessary to stand apart from other mobile utility services keeping in mind the problems the mobile industry was facing and make use of messaging as a communication tool due to its growing usage and popularity.
Messaging as a Communication Medium:
The main factor that drove Haptik’s breakthrough solution was the rise of Messaging as a Communication Medium. With the meteoric rise of Smartphone users in India with a majority comfortable with chat as a communication medium, it made sense to leverage that growth to boost engagement. High user engagement could be achieved through high-utility chatbots (to complete day-to-day tasks). This roster of pre-trained chatbots then needed to be bundled into an SDK that could be plugged into an app.
One of the major ways mobile engagement runs is through push notifications. However, as more and more brands start leveraging push notifications, their effectiveness lowers. The solution is personalisation. For instance, any user is more likely to click on a message that comes from a friend or a utility based message as opposed to an advertisement from a brand. However, the costs of building an in-app messenger are too high to justify the returns. In the same vein, all digital marketing efforts and engagement efforts (SMS, Social Media platforms) are slowly becoming ineffective as saturation rates hit an all-time high.
The only solution that can address all these issues at the same time is a chatbot. However, there are very few existing tools where a chatbot (or multiple) could easily plug in to an app and provide multiple services at once. Chatbots are also a fairly new entrant into the market purely as engagement tools.
In order to fulfill our clients requirements, Haptik had to build the following capabilities:
- An SDK that contains multiple chatbots that can be instantly embedded into any app or web client with a memory footprint under 1 MB.
- A chatbot with great utility: Our entire roster of chatbots includes over 40+ bots that offer everything from reminders to flight/cab bookings to bill payments to jokes. We will be talking about one of our most important use cases: Our ‘Reminders’ chatbot.
The chatbot converses with a user to understand their needs and then calls and reminds them about their specific task.
Haptik trained its models based on User Generated Data – where conversations that the users have is fed back to our algorithms so that they can improve with every conversation. A chat assistant can take over the chat when the bot is unable to handle it and this data is used to train the chatbot. For an idea of scale, Haptik had processed more than 0.65 billion messages till date from our network across platforms.
There’s an entire team of people dedicatedly responsible for generating new data every day. These roles can be broken down into 3 sub-areas Bot Builders: Making a bot end to end i.e. developing the character, tone, content, and responses of the bot. AI Bot Trainers: Monitoring user queries, labelling data that is unanswered and feeding it back into the data set for the bot to learn and respond to those queries over time and Bot Quality Analysts: Reviewing the bot from a qualitative perspective, understanding user requirements and relaying that feedback to the product management team to improve the experience. These Bot Quality Analysts are responsible for improving a bots capabilities by observing it’s behaviour, and improving its personality and quality over time. Their responses and suggestions are fed into the system and we have built tools for easy entry of data which is already annotated. Additionally, Wikipedia articles so that the bot could understand the basics of the English language.
A robust data pipeline helps understand what the user says and tries to find an answer for the user. To actually call a user once a reminder is set, an event-based architecture is set up – where a job is scheduled to run at the time of the reminder, so at the time of the reminder, Haptik tell the partner to call the user. When the user answers the call, TTS (Text-to-Speech) is applied to have a machine remind the user with the appropriate message.
How Does The Solution Work?
Once the user enters the channel, they can either tap a particular task or directly enter their reminder request as a message. Eg. Wake me up at 7 am. The chatbot asks for any details that are missing. Eg. On which days should I remind you? The reminder is set.
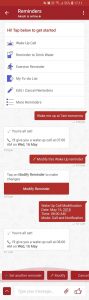
There are over 8 types of reminders.
- Wake-up Call
- Reminder to Drink Water
- Reminder to Call
- My To-do List
- Birthday reminder
- Medicine Reminder
- Exercise Reminder
- Meeting Reminder
At the specified time, the chatbot calls the user to remind them about their task. The bot reads out a personalized script greeting the user and reminds them to complete their task. The wake up calls are accompanied by a motivational quote as a notification. For To-Do reminders, the users are also asked to confirm whether they completed a task or wish to snooze it for a while.
Different AI Models Used:
A Hybrid Model: Haptik uses a hybrid model that combines a neural conversational model and a rule-based graph dialogue system that assists users in scheduling reminders through a chat conversation. The graph-based system has high precision and provides a grammatically accurate response but has a low recall.
The neural conversation model can cater to a variety of requests, as it generates the responses word by word as opposed to using canned responses. The hybrid system shows significant improvements over the existing baseline system of rule based approach and caters to complex queries with a domain-restricted neural model. Restricting the conversation topic and combination of graph based retrieval system with a neural generative model makes the final system robust enough for real world application with unexpected user responses.
Chatbot NER: During our journey at Haptik, we ended up building and customizing different machine learning modules specifically focused on building Chatbots on narrow domains and which are targeted at an end to end completion of a specific task. One such integral module is Chatbot NER (Named Entity Recognition). Chatbot NER is a heuristic based subtask of information extraction that uses several NLP techniques to extract necessary entities from chat interface. There are a number of NER’s available like Stanford NER, spaCy NER, etc. but none of these are designed specifically for building Chatbots. There are a lot of customizations required in existing NER’s which we did while building Chatbot NER. We then open sourced this module to be available to the developer community to build on.
Impact Of Haptik’s Chatbot On Business:
Quantitative Benefits:
- Users with the Personal Assistant embedded inside had a 60% higher retention than users who did not use the Personal Assistant on the client’s app.
- The average increase in impressions is higher by 35.4%. (based on screens per session)
- The average increase in engagement is higher by 31.6% (based on avg. session duration)
- The client is able to monetize chat-based ad campaigns that leverage their existing audience.
- Using our technology, chatbots can go from 50% to 95% and higher automation in terms of chat response. Very little to no manual intervention is required when automation levels are high. The chat response time to any message goes from 45-50 seconds minimum(when chat agents are involved) to >2 seconds
Qualitative benefits
- The client saw a notable increase in engagement rates and is looking to implement a similar function across their other platforms to improve engagement.
- The client saw a notable surge in positive feedback and responses on the app and the app stores.
- Seasonal, timely ad campaigns can now be served using this chat format(eg. IPL updates)
- The integrated SDK takes up less than 1MB and is now the second most used feature on the client’s app. The chatbot has been given major prominence right on the landing screen.
Innovation Quotient
Haptik is among the only companies building chatbots with a hybrid approach. Most of the companies build them using the basic retrieval based model, but the generative and neural conversation model that we use helps us cater to queries which the bot is usually not trained on. The bot goes back to ask the user a variation of their query if it does not understand a particular part of the request.
We are also one of the very few, if not only, companies offering a fully trained embeddable set of 40+ utility chatbots that can be easily plugged in to any app or mobile website. Conversational training data being extremely hard to find, we used the data created by our chat assistants manually over the course of 4 years to train our chatbots. Over time we upskilled our chat assistants to be Bot QA’s who hold complete responsibility of how a channel works. Their main focus is to improve automation numbers by making sure that the chatbot understands user responses.
We have dedicated tools and dashboards to build bots, analyse a bot’s performance, and a chat-agent interface which allows manual intervention if a chatbot is unable to respond to a user query. All of these tools were built completely from scratch in house.
Chatbot QA’s can improve bot capabilities over time using these tools:
By adding new responses/nodes: The QA’s first use the analytics dashboard to check the break messages (they can either be task related or casual responses.) based on the validity of the query and the use case of the bot. They then deploy these changes and run tests to see if these new changes work well.
Improving existing bot capabilities: The Bot QA’s go through previous conversations/chat logs on the bot builder tool to see where the bot was not able to recognise user responses and identifies the reason for the chat break. If the problem not rectifiable at the QA level, it is then escalated to the Machine Learning team. Ultimately, the bot goes through each and every break message and make sure that chat bot is able to handle such queries.
Insights Gained:
Generative models are still in research phase and it is hard to control how they work. Everyone expects the neural model to take care of all queries, but we instead use it to get the user back towards a retrieval flow rather than aim at completing the conversation.
In an interesting twist, our chatbot picked up Hindi despite being completely programmed in English. This happened due to Seq2Seq, one of the algorithms used in the programming of our bots. Since our chat agents intervene whenever a chatbot fails to reply to a user, the algorithm then goes through these failed chats, analyzes the human agent-user conversation and learns from the replies given. This interactive process lead to our chatbot learning from the conversations with users who chatted in Hindi (typed in Latin script) without any programming guidance whatsoever. As our automation levels increased, we upskilled our Chat Assistants to become Bot Trainers and Quality Analysts, a first in the industry.
This is part of our ongoing series Innovate2Transform, where we bring to you the leaders of the industry talk about the latest in innovation, technology and trends in their industry sectors.
If you want to contribute, write to sindhuja@nasscom.in or pritha@nasscom.in
For more updates, follow NASSCOM CoE IoT-DSAI on LinkedIn, Twitter, Facebook, and Youtube