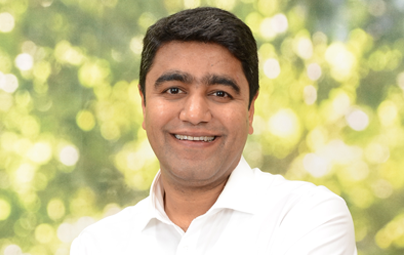
1. What is your take on Digital Transformation?
Digital Transformations today are AI-led and data-reliant. In this decade, this influence will only increase further as the scope expands immeasurably. Equally, we should bear in mind that the failure rates in digital transformation are high and some estimates have even put it at 70% even. Therefore, it’s imperative that we gather a deeper understanding of best practices.
Take a look around and see the kind of disruptions that are taking place today in practically every domain, as market leaders get ousted from their pristine position of glory. On one hand, you have the likes of GM being disrupted by Tesla and then again, who would have imagined how industries such as healthcare & wellness getting re-defined? Almost no one today is impervious to the forces – AI, Analytics, Cloud Computing, and other digital technologies. When financial gains do not come through, leaders may be demoralised to question if there’s any real value or is it plain hype?
In this regard, it’s important to remember a few things which I’d like to make a special mention of.
- It starts with Data Strategy but it should look beyond the technology aspect alone. For instance, the principles of data governance and data security will have to be considered. Whether the strategy is centralized or decentralized, is another area for a lookout. At the very outset, leaders need to develop a high level of consciousness, comprehend biases (if they exists) and how they impact the company’s data strategy as a whole.
<listyle=”text-align: justify;”>Then we come to the aspect of culture which can be segregated into two parts – the engineering aspect and talent.
Have we done enough to put processes and systems in place? Today, product development leverages Agile, DevOps and the likes to enable real-time communication, transparency with tremendous flexibility while effecting changes in double quick time. The monolithic engineering culture of the past may now be passe.
The talent culture is about hiring the right people and fostering an environment that supports continuous learning. Given the pace of change, reskilling will have to be a constant feature.
Most companies don’t seem to get this balance right – the combination of engineering and talent that drives the desired culture.
- The need to ask the right questions is of primary importance. How do you train the “engine” to give you the desired results? Keep feeding good quality data of course. On asking questions, let me give you an example. While acquiring new customers, it needs to be assessed whether they are really new or a transfer from a competitor? And, are the margins justifiable? Clear answers to questions such as these will define how the algorithms need to be designed which loops back to direction-setting for the company.
- Digital Transformation has to be a Boardroom conversation, else it’ll fall flat at some level. It has to be driven from the top. Are they putting their weight behind it; do they truly comprehend what it means, and similar questions need to be asked and answered. One needs the right kind of expertise at the Board level, otherwise, it will prove to be a severe bottleneck.
- Just because data is “free” doesn’t really mean it really is. There are ethical and legal bindings on how data can be used. A breach can result in severe reputational damage. Comprehending risk is the first step forward towards its mitigation. Being digital is also about understanding the element of risk involved.
2. For a company that is new to AI and aspires to jump on the wagon. What would your advice be?
Actully, the way I see it is that every company today irrespective of size is focussed on the Returns on AI. Far too easily people get swayed by Moonshots and admittedly, the glamour quotient is very high there. In reality, though, it’s the boring use cases that are sometimes more valuable in the digital transformation journey. For instance, how do you leverage AI to mitigate cybersecurity threats? The returns are massive if you are able to do a good job there but not necessarily the kind that makes headlines, right? That’s why I call them boring. Seek out the “boring” problems and solve them.- Startups need to be able to sell to Board members and CEOs. Beyond technology, they need to clearly articulate the business case with equal aplomb. Without this, conversations will not sustain.
- Thirdly, we need to focus o the AI + Human angle and not be carried away by sci-fi like proposition. After all, it has to be about augmenting human productivity in an environment where bots and humans can work alongside.
3. Please share your thoughts on how Data & Analytics are being used to create value which wasn’t possible earlier?
Possibly, the fintech example in India is a very good example of how “newbies” can disrupt a traditional industry such as banking and compel incumbents to follow suit to adopt emerging technology in their delivery. And really who could have imagined? Analytics, while combining data avalibility, is solving real world problems to carve out solutions that serve millions of customers.
If I give you 5 million songs in a drive, will you be able to replicate the Spotify model? Not really. Simply having access to data is not sufficient. Algorithms are designed in a manner to leverage data and serve the specific needs of customers. With time and better quality data, the quality of output improves exponentially too. This is how the EXPERIENCE economy works and the bar gets raised. Analytics is the backbone of Machine Learning and diverse industries such as healthcare, transportation, agriculture among others, are using these tools to disrupt the landscape and create niche markets as well.
4. What are your thoughts specifically on AI? Please distil reality from hype.
Hype is created from headlines and without a deeper understanding of things. For instance, a CEO reads up something and is greatly impressed by the idea, she forwards it to the team lead with a diktat to replicate it. Obviously, without having done a deep-down on feasibility. The team gets cracking and soon enough the results are disastrous which leads to an early abortion of the project. Things fall apart and only go downhill from there, careers are smashed and a feeling of despondency sets in.
Secondly, how is data being collected and used? Is it being used explicitly for the purpose stated?
Thirdly, is the AI engine being trained adequately to manage clients’ needs, provide support, handle delays in equipment usage and improve the overall SCM? These are only a few use cases that give you the returns and may be worthwhile to focus on, for starters.
Fourthly, and I’d like to reiterate – please focus on the “boring” jobs because that’s what will give you the desired value.
5. What are the challenges that technology should solve for India, giving precedence over others and how can AI help solve these from an Indian standpoint?
- Clearly, Fintech is a big area. It can play a very big role in formalising the economy. Developed countries leverage fintechs differently and it may not be a great idea to go after the very same models. For instance, in India, micro-lending is a strong use case and can be expanded further which will bring in a high degree of inclusivity.
- Social issues. For example, AI can be used to create identities that can help to trace missing people. It can also be used to control crime.
- Then we have the shared economy. This is creating immense value.
- Healthcare – use cases in diagnostics, electronic health records, predictive healthcare management are some powerful examples that can transform India.
6. What are the risks involved with AI and should it be regulated? If yes, how?
Increasingly, global leaders are coming out in the open and insisting that AI needs to be regulated too. To be sure there are issues pertaining to data manipulation, ethics, discrimination, unconscious bias and a host of others. These are human issues that get intractably linked to the “logic” of the algorithms. They can all lead to risks at various levels – individual, social, enterprise-wide and finally national even. We may even be looking at a situation where data is included as a fundamental right in the future. We need to understand the accountability of humans in this chain in greater depth.
The challenge of having it regulated too early is to do with innovation. Too much of a constricting framework will be detrimental to the spirit of innovation. At the same time, a lax structure puts mankind at great risk. So, the future is about striking the balance. Let me give you a classic example of an ethical dilemma that we are yet to resolve. If an autonomous car is involved in a crash how will responsibility and accountability be apportioned between the software maker and the car manufacturer? Digital transformation, at one level, will keep throwing at us newer challenges to do with ethics.
7. We keep talking about innovation. From a policy standpoint, what does a country really need to democratize innovation? What would it take for many more innovators to come forward and monetize their ideas?
As I said earlier, we need a balance between open systems and control. Do it too early and you risk nipping innovation at the bud. Do it too late and the consequences can be catastrophic. Genuine mistakes are part of the machine learning process and will have to be treated that way.
- We need policies to address open data, open APIs, open platforms and the likes.
- We can’t have policies for standalone companies. In an ecosystem-led environment, policies will have to be inclusive to impact all the stakeholders in a positive manner – well, at least as far as possible.
8.Your leadership mantra in the deep-tech age and pivots for the future, please?
- Do not jump on the AI bandwagon without having done the due diligence – skills, culture, hard questions, buy-in from the Board, etc.
- Boring stuff will get you the results.
- Risk assessment – ethical, legal, etc. – needs to be done early.
- Technology augments human productivity. Don’t start with the goal of replacing humans with machines.