From Generative to Agentic: A Comparative Analysis of AI Models
“The evolution from generative to agentic AI systems, comparing their architectural frameworks, operational capabilities, and strategic business applications. The comparison reveals how agentic AI’s autonomous decision-making capabilities address fundamental limitations of generative models while creating new implementation challenges for enterprise adoption.”
Artificial Intelligence (AI) has undergone significant advancements in recent years, evolving from rule-based systems to sophisticated neural architectures capable of performing increasingly complex functions. This evolution has led to the emergence of two distinct AI paradigms that are transforming enterprise technology strategies: generative AI and agentic AI. Generative AI has garnered considerable attention for its ability to create content across various formats, while agentic AI represents the next frontier in autonomous systems capable of independent decision-making and task execution.
For executive leadership and technology strategists, understanding the technical distinctions between these AI models is crucial for effective implementation and maintaining a competitive edge. This comparative analysis examines the underlying architectures, operational capabilities, and strategic applications of both generative and agentic AI systems. We will evaluate their respective strengths, limitations, and strategic implications for enterprises seeking to leverage these technologies for business transformation.
As organisations continue to navigate the complexities of digital transformation, the selection and deployment of appropriate AI models represent critical decision points with far-reaching implications for operational efficiency, innovation capacity, and market competitiveness. This analysis aims to provide technical decision-makers with a framework for evaluating these technologies against specific business requirements and strategic objectives.
Understanding Generative AI
Generative AI encompasses a class of machine learning models designed to learn data distributions and generate new content that resembles training examples without explicit programming. These systems typically employ deep neural network architectures, including Generative Adversarial Networks (GANs), Variational Autoencoders (VAEs), and transformer-based models like GPT (Generative Pre-trained Transformer). The fundamental principle underlying these models is their ability to identify and replicate patterns in vast datasets, enabling them to produce contextually appropriate outputs across various domains and formats. The technical architecture of generative AI models involves sophisticated training methodologies, including unsupervised and semi-supervised learning approaches. These models process enormous volumes of data—often measured in trillions of parameters—to establish complex correlations and patterns. Generative AI can reduce workload by 60% to 70%, making it a powerful tool for enhancing productivity and innovation. [1]
For instance, large language models (LLMs) are trained on diverse text corpora to develop capabilities for natural language understanding and generation. Similarly, diffusion models like DALL-E and Stable Diffusion utilise iterative denoising processes to generate high-fidelity images from textual descriptions. Bill Gates, Co-Founder of Microsoft, has remarked, “Generative AI has the potential to change the world in ways that we can’t even imagine. It has the power to create new ideas, products, and services that will make our lives easier, more productive, and more creative”.
Strategically, generative AI has demonstrated significant value in domains requiring creative content production, language translation, and data augmentation. Organisations have deployed these models to automate content creation workflows, enhance customer service through sophisticated conversational agents, and accelerate product development through generative design. Over 60% of companies are already using generative AI in the workplace, highlighting its widespread adoption and impact. [1]
However, these systems operate within defined parameters and typically lack autonomous decision-making capabilities, requiring substantial human oversight to ensure outputs align with business objectives and quality standards. Sam Altman, CEO of OpenAI, has noted, “I think AI is going to be the greatest force for economic empowerment and a lot of people getting rich we have ever seen”. This underscores the importance of strategic oversight in the deployment of generative AI to harness its full potential while mitigating risks.
The Rise of Agentic AI
Agentic AI represents a significant advancement beyond generative systems, characterised by its capacity for autonomous decision-making and goal-oriented behaviour without continuous human direction. These systems integrate multiple AI components—perception, reasoning, planning, and execution—into unified architectures capable of understanding contexts, formulating strategies, and executing complex tasks independently. The core technical distinction of agentic AI lies in its deliberate action framework: these systems can evaluate options, select optimal approaches, and adapt strategies based on evolving environments. By 2025, it is estimated that 75% of large enterprises will use agentic AI for operational decision-making.[2]
The technical architecture of agentic systems typically incorporates reinforcement learning frameworks, knowledge graphs, and sophisticated planning algorithms that enable systematic reasoning. Unlike generative models that primarily respond to prompts, agentic AI employs episodic memory and causal reasoning to maintain context continuity across multiple interactions and task sequences. The market value of agentic AI is projected to grow from USD 5.1 billion in 2024 to over USD 47 billion by 2030, with a compound annual growth rate (CAGR) of over 44%.[3] This enables persistent state management and objective tracking—capabilities essential for autonomous operation in dynamic environments. Implementation frameworks such as Hierarchical Task Networks (HTNs) and Multi-Agent Reinforcement Learning (MARL) provide the computational foundation for these capabilities.
Andrew Ng, Co-founder of Coursera and Adjunct Professor at Stanford University, has highlighted the transformative potential of agentic AI: “The ability of AI to make autonomous decisions and adapt to new information in real-time is a game-changer for industries that rely on complex decision-making processes”. This sentiment is reflected in the growing adoption of agentic AI across various sectors. Companies implementing agentic AI have reported up to a 30% increase in operational efficiency.[4]
In enterprise contexts, agentic AI demonstrates value in scenarios requiring continuous operational oversight, complex decision sequences, and adaptive responses to changing conditions. Financial institutions have deployed agentic systems for algorithmic trading and risk management, leveraging their ability to process market signals and execute transactions autonomously. Similarly, manufacturing organisations have implemented agentic AI for supply chain optimisation, where systems continuously monitor inventory levels, production capacities, and market demands to coordinate logistics operations efficiently. These applications highlight agentic AI’s strategic advantage in domains where autonomous decision-making capabilities directly translate to operational efficiency and competitive differentiation.
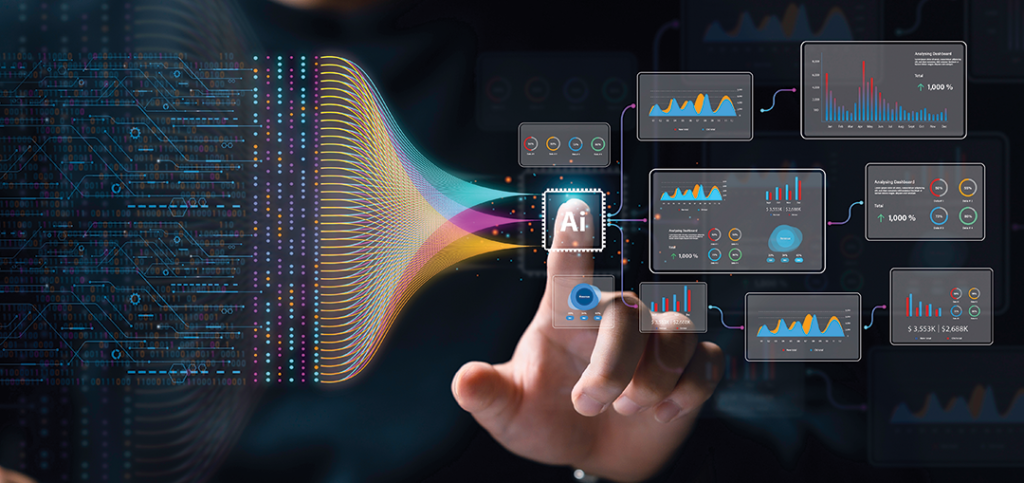
The Imperative for Reinvention in Modern Organisations
Contemporary organisations face unprecedented challenges in maintaining relevance within rapidly evolving technological landscapes. Customer expectations have transformed dramatically, demanding personalised, instantaneous, and intelligent interactions across multiple touchpoints. Traditional operational models are increasingly insufficient for addressing these complex requirements, creating a critical need for strategic technological reinvention.
Generative AI emerges as a strategic mechanism for digital transformation, offering organisations sophisticated tools to reimagine their operational architectures. By integrating intelligent systems that can dynamically adapt, learn, and generate contextually relevant solutions, enterprises can develop more agile, responsive, and intelligent organisational frameworks. This technological integration transcends mere automation, representing a fundamental restructuring of strategic capabilities.
Leadership plays a pivotal role in driving AI-powered change. Successful GenAI implementation requires visionary executives who can navigate complex technological landscapes, balance innovation with risk management, and cultivate a culture of continuous learning and technological adaptation. Strategic leaders must develop comprehensive AI roadmaps that align technological investments with broader organisational objectives, ensuring sustainable and meaningful digital transformation.
Key Differences Between Generative AI and Agentic AI
Understanding the distinctions between generative AI and agentic AI is crucial for leveraging these technologies effectively. Below is a comparative analysis of their key features:
Feature
|
Generative AI
|
Agentic AI
|
Primary Function
|
Content Generation
|
Autonomous Decision-Making
|
Learning Mechanism
|
Pattern Recognition (LLMs, GANs, Diffusion Models)
|
Reinforcement Learning, Planning, Adaptation
|
User Dependency
|
Requires user input (prompts)
|
Operates independently with real-time data
|
Applications
|
Text, image, video, music generation
|
Robotics, cybersecurity, supply chain management
|
Adaptability
|
Limited to learned patterns
|
Continuously evolves through real-world interactions
|
Decision-Making
|
Predictive, but not autonomous
|
Proactive and goal-oriented
|
Ethical Concerns
|
Misinformation, deepfakes, bias
|
Transparency, accountability, control
|
Detailed Analysis
- Primary Function: Generative AI focuses on creating content such as text, images, videos, and music. It excels in tasks that require creativity and content generation. In contrast, agentic AI is designed for autonomous decision-making, enabling it to perform tasks and make choices without human intervention.
- Learning Mechanism: Generative AI models, such as Large Language Models (LLMs), Generative Adversarial Networks (GANs), and diffusion models, rely on pattern recognition to generate new content. Agentic AI, on the other hand, employs reinforcement learning, planning, and adaptation to make decisions and execute tasks autonomously.
- User Dependency: Generative AI requires user input in the form of prompts to generate content. It reacts to the data it has been trained on and produces outputs based on that input. Agentic AI operates independently, using real-time data to make decisions and adapt to changing environments.
- Applications: Generative AI is widely used in applications such as text, image, video, and music generation. It is valuable for automating content creation workflows and enhancing customer engagement. Agentic AI finds applications in robotics, cybersecurity, and supply chain management, where autonomous decision-making and task execution are critical.
- Adaptability: Generative AI is limited to the patterns it has learned from its training data. It can generate content based on these patterns but does not adapt beyond them. Agentic AI continuously evolves through real-world interactions, learning from new data and experiences to improve its decision-making capabilities.
- Decision-Making: Generative AI is predictive but not autonomous. It generates content based on learned patterns but does not make decisions independently. Agentic AI is proactive and goal-oriented, capable of making autonomous decisions and adapting strategies based on evolving environments.
- Ethical Concerns: Generative AI raises ethical concerns related to misinformation, deepfakes, and bias in generated content. Ensuring the authenticity and fairness of its outputs is a significant challenge. Agentic AI's ethical concerns revolve around transparency, accountability, and control, as these systems make autonomous decisions that can have far-reaching implications.
Strategic Implications for Businesses
- 1. Choosing the Right AI Model
Organisations must carefully assess their specific needs before implementing AI technologies. Generative AI is ideal for businesses that require automated content creation and data augmentation. In contrast, agentic AI is best suited for operations that demand autonomous decision-making and adaptability.
- 2. Combining Generative and Agentic AI
Hybrid AI systems that integrate both models can offer enhanced capabilities. For example:
- Intelligent Virtual Assistants: Combining generative AI’s language processing with agentic AI’s decision-making can create advanced AI-driven customer service agents.
- AI in Healthcare: Generative AI can analyse medical scans, while agentic AI can autonomously manage patient monitoring and treatment recommendations.
- Financial Fraud Detection: Generative AI can generate risk models, while agentic AI can take real-time actions to prevent fraud.
- 3. Ethical Considerations
Businesses adopting AI technologies must prioritise ethical AI development. This includes:
- Bias Mitigation: Ensuring training datasets are diverse and free from biases.
- Transparency: Making AI decision-making processes explainable and auditable.
- Security Measures: Implementing safeguards to prevent AI misuse.
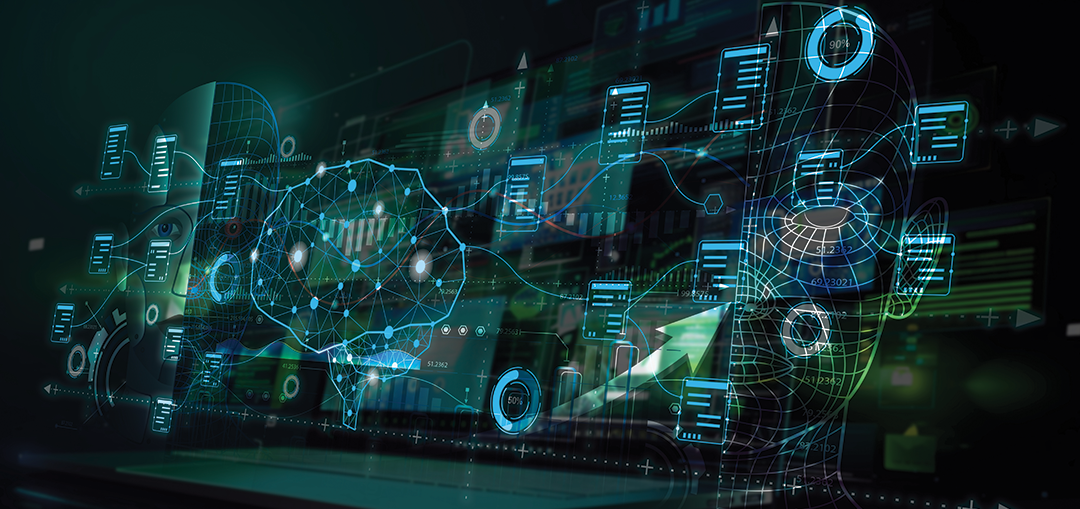
Emerging Trends
Technical advancements in both generative and agentic AI are accelerating, with several significant trends reshaping implementation approaches and capability boundaries.
- Generative AI Trends
In generative AI, there is an increasing emphasis on multimodal architectures that seamlessly integrate text, image, audio, and video processing capabilities within unified models. These developments have enabled more sophisticated content generation applications, including systems capable of producing coherent multimedia narratives from textual descriptions. Additionally, techniques like constitutional AI and reinforcement learning from human feedback (RLHF) are addressing alignment challenges by enabling more precise control over model outputs and behaviour.
- Agentic AI Trends
For agentic AI, emerging trends focus on enhanced reasoning capabilities and collaborative frameworks. Developments in symbolic reasoning integration with neural architectures are producing systems with improved logical consistency and transparency in decision processes. Multi-agent frameworks represent another significant advancement, enabling coordinated operation of specialised AI systems to address complex challenges collectively. These frameworks demonstrate promise for enterprise applications requiring diverse expertise domains, such as integrated business planning or comprehensive cybersecurity operations.
- Convergence of Generative and Agentic AI
The convergence of generative and agentic capabilities represents perhaps the most significant technical trend. Emerging systems leverage generative components for content creation while employing agentic frameworks for process coordination and decision execution. This integration enables applications like autonomous research assistants that can independently formulate questions, gather information, synthesise findings, and present coherent analyses. Similarly, emerging product development platforms leverage generative design capabilities within agentic frameworks that systematically evaluate options against engineering constraints and business requirements. These hybrid approaches demonstrate how complementary AI architectures can address limitations inherent in individual paradigms while creating new implementation possibilities for enterprise applications.
Examples:
- Intelligent Virtual Assistants: Combining generative AI’s language processing with agentic AI’s decision-making can create advanced AI-driven customer service agents.
- AI in Healthcare: Generative AI can analyse medical scans, while agentic AI can autonomously manage patient monitoring and treatment recommendations.
- Financial Fraud Detection: Generative AI can generate risk models, while agentic AI can take real-time actions to prevent fraud.
Ethical Implications and Challenges
The technical architectures of generative and agentic AI systems present distinct ethical challenges that require tailored governance frameworks.
-
- 1. Generative AI
Generative AI raises significant concerns regarding intellectual property rights, output authenticity, and potential misuse for misinformation propagation. These issues stem from fundamental training methodologies that involve learning from copyrighted materials without explicit permission and the systems’ capacity to produce convincing but potentially misleading content.
Technical Mitigation Approaches:
- Watermarking Solutions: Embedding detectable signatures within AI-generated content to ensure authenticity.
- Provenance Tracking Mechanisms: Maintaining comprehensive records of content origins and modification histories to verify the source and integrity of generated outputs.
-
- 2. Agentic AI
Agentic AI introduces more complex ethical considerations related to decision transparency, operational boundaries, and responsible autonomy. The multi-component architectures and sophisticated decision processes of these systems often function as “black boxes,” making it challenging to audit reasoning processes or attribute responsibility for outcomes.
Technical Approaches:
- Explainable AI Methods: Providing human-interpretable justifications for system decisions to enhance transparency.
- Formal Verification Techniques: Mathematically proving system behaviour within defined constraints to ensure reliability.
- Containment Frameworks: Establishing explicit operational boundaries to ensure safe deployment in sensitive domains.
-
- 2. Common Challenges
Both AI paradigms share common challenges regarding data privacy, bias mitigation, and appropriate oversight mechanisms. Enterprises implementing these technologies must develop comprehensive governance frameworks addressing technical safeguards, operational policies, and compliance monitoring.
Governance Frameworks:
- Technical Safeguards: Implementing robust security measures to protect data privacy and prevent misuse.
- Operational Policies: Establishing clear guidelines for the ethical use of AI technologies.
- Compliance Monitoring: Continuously evaluating AI systems to detect emergent biases or unintended behaviours as they evolve through continuous learning.
Additionally, organisations must establish clear accountability structures that define responsibility allocation between human operators and AI systems, particularly for agentic applications where autonomous decision-making capabilities may obscure traditional responsibility boundaries.
These ethical considerations are crucial for ensuring the responsible development and deployment of AI technologies, safeguarding both organisational interests and societal values.
The Road Ahead for AI
The technical trajectories for generative and agentic AI suggest continued advancements along several dimensions, with significant implications for enterprise applications and competitive landscapes.
- 1. Generative AI Advancements
For generative AI, we anticipate architectural improvements that will enable more efficient operation with reduced computational requirements, making sophisticated capabilities accessible to a broader range of organisational contexts. Advances in controllability mechanisms are also expected to enable more precise output specification while maintaining generation quality, addressing current limitations in consistency and contextual appropriateness.
- 2. Agentic AI Developments
Agentic AI development appears focused on enhanced reasoning capabilities and expanded operational domains. Research in causal reasoning, analogical thinking, and counterfactual analysis promises more sophisticated planning and decision-making capabilities applicable to complex enterprise challenges. Additionally, advancements in safe exploration techniques will likely enable agentic systems to operate effectively in increasingly sensitive domains while maintaining appropriate risk boundaries.
- 3. Cross-Paradigm Integration
Cross-paradigm integration represents a particularly promising direction, with hybrid systems leveraging complementary capabilities across generative and agentic domains. These integrations may enable new application categories, including fully autonomous business process execution with minimal human supervision and intelligent systems capable of identifying organisational challenges and independently developing solutions.
Examples
- Autonomous Research Assistants: Combining generative AI for content creation with agentic AI for process coordination and decision execution.
- Product Development Platforms: Leveraging generative design capabilities within agentic frameworks to systematically evaluate options against engineering constraints and business requirements.
For enterprises, these developments suggest opportunities for substantial operational transformation and competitive differentiation through early adoption and integration of advanced AI capabilities. However, realising these benefits will require deliberate technical infrastructure development, talent acquisition strategies, and governance frameworks that balance innovation with appropriate safeguards.
Strategic Implications:
- Operational Transformation: Early adopters of hybrid AI systems can achieve significant efficiency gains and innovation.
- Competitive Differentiation: Integrating advanced AI capabilities can provide a competitive edge in rapidly evolving markets.
Conclusion
The comparative analysis of generative and agentic AI highlights their distinct technical architectures and complementary capabilities. Generative AI excels in content creation and pattern recognition, making it valuable for applications requiring sophisticated information synthesis and creative outputs. In contrast, agentic AI demonstrates superior capabilities in autonomous decision-making and task execution, addressing limitations inherent in reactive systems.
As these technologies evolve, organisations must make critical decisions regarding adoption strategies, integration approaches, and governance frameworks. Technical leaders should develop comprehensive AI strategies that consider both immediate implementation opportunities and longer-term capability development. These strategies should address infrastructure requirements, talent development needs, and appropriate governance mechanisms tailored to specific AI paradigms and application domains.
About the author :
Dr. Bishan Chauhan, Head – Cloud Services & AI / ML Practice, Motherson Technology Services
Comment
Gen AI Is really Involving fast