Next-Generation Sequencing (NGS), a major advancement in the field of genomics research, has completely changed how we think about genetics and molecular biology. This technology has made it possible to decode entire genomes, examine gene expression, and delve into the intricate workings of cellular processes. Along with these amazing developments come difficulties in digesting and gleaning useful information from the enormous amount of data produced by NGS. Enter data analytics—the unsung hero that solves the genetic puzzles buried in the data's strands. We will examine the dynamic interaction between NGS and data analytics in this blog, emphasizing how merging both disciplines enables researchers to discover the mysteries of life.
Fundamentals of Next-Generation Sequencing
Genomic research has entered a previously inconceivable realm of precision and depth because of NGS technologies. Illumina, Oxford Nanopore, and PacBio are just a few of the platforms that have democratized genome sequencing and made it more accessible and affordable. Sample preparation, sequencing, and data creation are part of the NGS workflow, which enables researchers to examine DNA, RNA, and epigenetic changes. Applications for this adaptability include tracing the evolution of species and researching rare genetic disorders.
Data Landscape of Next-Generation Sequencing
The amount of data generated by NGS is astounding. A single human genome sequencing run is thought to produce gigabytes of raw data. Short reads, long reads, quality scores, and base calling are the main features of this data avalanche. In the analysis pipeline, the raw data is frequently saved in formats like FASTQ, BAM, and VCF, each of which has a particular function.
Challenges in NGS Data Analysis
NGS data processing and interpretation present numerous challenges. To guarantee proper downstream analysis, data preprocessing includes quality check, adaptor removal, and trimming. To account for genetic differences, alignment and mapping against reference genomes require complex algorithms. Careful filtering is necessary for variant calling that detects Single Nucleotide Polymorphisms (SNPs) and Insertions/Deletions (INDELs), in order to reduce false positives and negatives. The intricacy of transcriptomics is further increased by the measurement of gene expression levels and the detection of alternative splicing processes.
Data Analytics Techniques for NGS
1. Exploratory Data Analysis (EDA)
Researchers frequently start with Exploratory Data Analysis (EDA) to learn more about their NGS datasets before moving on to more involved studies. Making visuals, providing summary statistics, and spotting patterns or abnormalities in the data are parts of the EDA process. Understanding the distribution of quality ratings, spotting potential batch effects, and locating outliers that can affect further analysis are aided by this stage.
2. Methods of Machine Learning
Due to its capacity to identify intricate patterns within big datasets, machine learning has found extensive use in NGS data processing. Classification algorithms are used to forecast outcomes, such as locating genetic variations linked to a disease. To model quantitative relationships, such as forecasting gene expression levels based on different variables, regression techniques can be used. Using clustering techniques, similar data points are grouped together to help identify genes with differential expressions or separate samples based on their expression profiles.
3. Deep Learning Applications
Due to its capacity to extract complex patterns from big datasets, deep learning, a type of machine learning, is especially well suited for NGS data. Images produced by long-read sequencing devices like Oxford Nanopore are processed using Convolutional Neural Networks (CNNs). These networks can improve base-calling precision and aid in read-sequencing error correction. Utilizing their capacity to spot sequential dependencies in data, RNN - Recurrent Neural Networks and LSTM - Long Short-Term Memory networks are used for tasks including variant prediction and read alignment.
4. Network Analysis
The interactions of genes, proteins, and other biological components are frequently the subject of NGS data. Graph theory and other network analysis approaches aid the understanding of these intricate relationships. Gene co-expression and functional relationships are highlighted by gene interaction networks. Enhancing our comprehension of the underlying biology is supported by the identification of important molecular pathways that are impacted by certain circumstances through pathway analysis. Insights into the landscape of gene regulation are provided by regulatory networks that reveal the complex web of connections between transcription factors, genes, and other regulatory components.
5. Time-Series Analysis
NGS data analytics can be applied to studies that employ time-series data or dynamic processes to track changes over time. This is particularly important in transcriptomics, where researchers are trying to understand how patterns of gene expression change over time. Techniques for time-series analysis, such as dynamic Bayesian networks or autoregressive models, can show how temporal variables affect gene expression profiles.
6. Data Integration from Multiple Omics
Researchers frequently combine multi-omics data from genomes, transcriptomics, proteomics, and other fields to develop a thorough knowledge of biological systems. With the use of data integration tools, hidden linkages and interactions between several levels of molecular information can be revealed. Researchers can create more precise and comprehensive models of biological processes and disease pathways by combining these datasets.
The foundation upon which the potential of Next-Generation Sequencing data is leveraged is data analytics techniques. Using these methods, researchers may study the dynamics of gene expression, decipher genetic variants and the complex networks that control biological functions. Data analytics opens opportunities to understanding the secrets hidden in DNA, ranging from straightforward exploratory analysis to cutting-edge machine learning and deep learning techniques. The synergy between data analytics and genomics is likely to continue defining the future of biology and propel developments in personalized medicine, biotechnology, and other fields as NGS develops and generates more and more data.
Tools and Software for NGS Data Analytics
The analysis of NGS data uses a variety of hardware and software. Basic functionalities for alignment, variant calling, and other tasks are provided by bioinformatics mainstays including BWA, SAMtools, and GATK. Workflow pipelines that provide repeatability and scalability, such Nextflow and Snakemake, streamline the analytic process. Researchers without substantial programming experience can access interfaces on user-friendly platforms like Galaxy and BaseSpace. Researchers can create customized studies that are targeted to their queries, thanks to programming languages like Python and R, and specialized libraries.
Real-world Applications
NGS data analytics have an impact on many different fields. NGS in clinical genomics provides quick diagnosis, individualized treatment, and the discovery of genetic variations linked to a disease. NGS is used by evolutionary biologists to investigate phylogenetics, solve population genetic puzzles, and identify cross-species adaptations. Researchers in functional genomics decipher complex gene expression networks, epigenetic changes, and regulatory elements. Additionally, NGS enables metagenomics that allows us to comprehend microbial communities and unearth environmental DNA treasures.
Future Directions and Challenges
The future of NGS data analytics is full of both opportunities and obstacles. Scalability and big data solutions are essential as the amount of data increases dramatically. A comprehensive understanding of cellular processes will be possible with the integration of multi-omics data, including genomes and proteomics. As NGS data becomes more integrated and available, ethical considerations including privacy, data sharing, and informed permission will become increasingly important. Real-time analysis and long-read sequencing enhancements, for example, have the potential to further expand our understanding of genomics.
Bottomline
Next-Generation Sequencing has served as the compass and data analytics has served as the map in the quest to unlock the mysteries of life hidden inside the DNA. By enabling us to decode genomes, understand hereditary illnesses, and explore the complex web of molecular connections, they have advanced the boundaries of biological discovery. The symbiosis between NGS and data analytics promises to change our understanding of biology and the future of medicine, agriculture, and beyond as we stand on the verge of extraordinary achievements. The relationship between NGS and data analytics will continue to influence the field of genomics research for many years to come through constant innovation, cooperation, and discovery.
About the Author
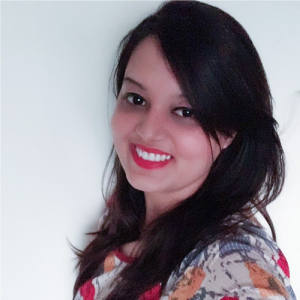
Purva Shah
Purva is a Product Marketing Manager at eInfochips, specializing in Medical Device Practice. With a background in engineering and marketing, she combines technical expertise with strategic thinking. Purva's role involves defining product strategies, identifying market opportunities, and ensuring customer-centric innovation in healthcare technology. She carries 7+ years of experience in Product Positioning, Practice Marketing, Go-To-Market Strategies, and Solution Consulting.